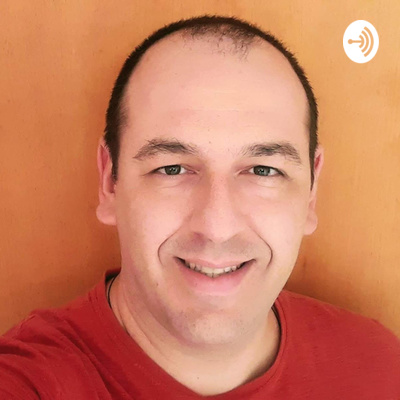
Thales Sehn Körting
By Thales
Enjoy!
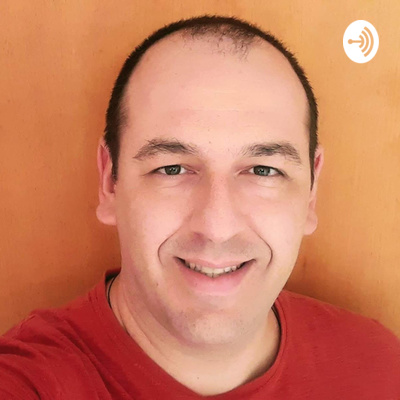
Thales Sehn KörtingDec 11, 2020
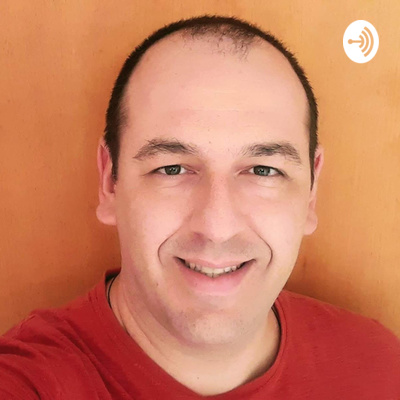
What is Data Science? (Part 1)
Follow my podcast: anchor.fm/tkorting
Subscribe to my YouTube channel: youtube.com/tkorting
The intro and the final sounds were recorded at my home, using an old clock that belonged to my grandmother.
Thanks for listening
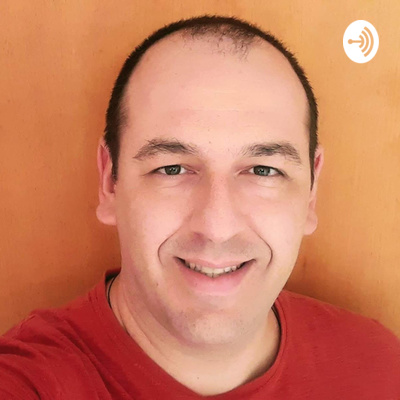
Is Deep Learning FAIR?
Deep Learning articles use benchmarks to measure the quality of the results. However, several benchmarks do not have the copyright of all data used. So, how to believe that every paper uses the same benchmark?
From https://www.go-fair.org/fair-principles/ we have the description of the FAIR acronym
- Findable: The first step in (re)using data is to find them. Metadata and data should be easy to find for both humans and computers.
- Accessible: Once the user finds the required data, she/he needs to know how can they be accessed, possibly including authentication and authorisation.
- Interoperable: The data usually need to be integrated with other data. In addition, the data need to interoperate with applications or workflows for analysis, storage, and processing.
- Reusable: The ultimate goal of FAIR is to optimise the reuse of data. To achieve this, metadata and data should be well-described so that they can be replicated and/or combined in different settings.
From the article Implementing FAIR Data Principles: The Role of Libraries (https://libereurope.eu/wp-content/uploads/2017/12/LIBER-FAIR-Data.pdf) we include the following additional description on the Reusable term: Data and collections have a clear usage licenses and provide accurate information on provenance.
Top-3 dataset for Deep Learning, based on a 25 list (https://www.analyticsvidhya.com/blog/2018/03/comprehensive-collection-deep-learning-datasets/)
- From http://cocodataset.org/#termsofuse: The COCO Consortium does not own the copyright of the images.
- From http://image-net.org/download-faq: The images in their original resolutions may be subject to copyright, so we do not make them publicly available on our server.
- From https://storage.googleapis.com/openimages: While we tried to identify images that are licensed under a Creative Commons Attribution license, we make no representations or warranties regarding the license status of each image and you should verify the license for each image yourself.
Follow my podcast: http://anchor.fm/tkorting
Subscribe to my YouTube channel: http://youtube.com/tkorting
The intro and the final sounds were recorded at my home, using an old clock that belonged to my grandmother.
Thanks for listening
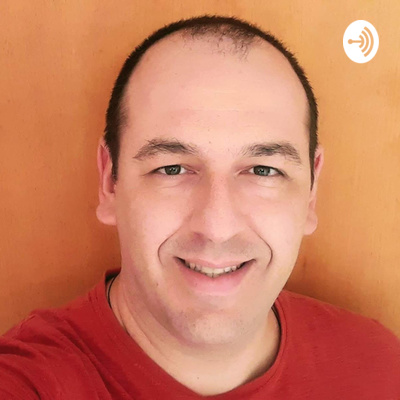
Do you trust in pretrained Deep Learning models?
Several authors rely on transfer learning from pretrained models, arguing that using well-known datasets, which are available on the internet (e.g. ImageNet) their model will be able to handle a specific problem with a reduced training step.
In Remote Sensing this perspective is also becoming a trend when using Deep Learning techniques to classify Remote Sensing datasets.
In my opinion, the datasets used for pretrain are very different from Remote Sensing targets, mainly in two aspects:
- spatial resolution: a sensor can be ultra high spatial resolution (50cm for example) or very low resolution (2km for a single pixel), and the edges in all these images are different
- spectral resolution: the datasets found on the internet are composed by color pictures, obtained mainly by phone cameras, which are composed by 3 channels (red, green and blue). In Remote Sensing we can have several spectral channels, such as yellow or red-edge bands (available in WorldView-2), or infra-red channels, available in most of the satellites. How to train a model using 3 bands, when in reality you can have at least 5 bands with so different information?
If you agree, or if you do not agree, please give some feedback and let's learn together.
Follow my podcast: http://anchor.fm/tkorting
Subscribe to my YouTube channel: http://youtube.com/tkorting
The intro and the final sounds were recorded at my home, using an old clock that belonged to my grandmother.
Thanks for listening
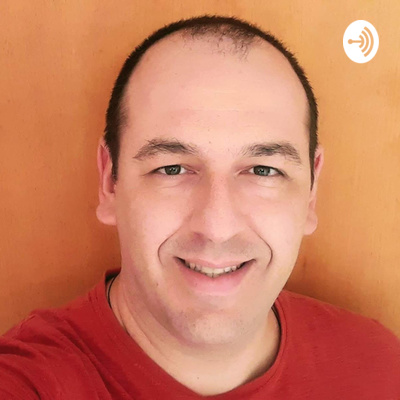
Are you sure you apply only Data Mining to your database?
In this podcast I discuss the (sometimes) wrong use of the term Data Mining, with in accord to the paper
From Data Mining to Knowledge Discovery in Databases, written in 1996 by Usama Fayyad, Gregory Shapiro, and Padhraic Smyth,
is defined as: Data mining is a step in the KDD process that consists of applying data analysis and discovery algorithms that produce a particular enumeration of patterns (or models) over the data.
KDD means Knowledge Discovery in Databases, and is composed by the following steps:
Data -> (selection) -> Target Data -> (preprocessing) -> Preprocessed Data -> (transformation) -> Transformed Data -> (data mining) -> Patterns -> (interpretation/evaluation) -> Knowledge
Several authors call Data Mining when they are performing the entire cycle (from Data to Knowledge) and not only the data mining step, which can be represented also by the use of classification/clustering algorithms.
The reference paper is available at: https://wvvw.aaai.org/ojs/index.php/aimagazine/article/download/1230/1131
Follow my podcast: http://anchor.fm/tkorting
Subscribe to my YouTube channel: http://youtube.com/tkorting
The intro and the final sounds were recorded at my home, using an old clock that belonged to my grandmother.
Thanks for listening
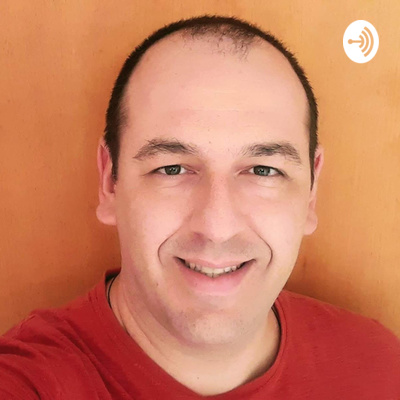
When the high resolution is not so high...
In this podcast I discuss the wrong use of the term Resolution in scientific articles or in the general media. Resolution in Remote Sensing can be used to describe several aspects of images, such as:
- temporal resolution: the time difference between two images of the same place
- spectral resolution: related to the number of bands and wavelengths, such as in Panchromatic, Multispectral, Hyperspectral, or Ultraspectral
- radiometric resolution: the number of bits needed to store a pixel value (e.g. 8 bits in Landsat 7 or 11 bits in WorldView-2)
- spatial resolution: the focus of this podcast, relating the area represented by a single pixel in an image
I provide an interesting reference with an easy to use table, to understand what can be considered High Spatial Resolution, or Low Spatial Resolution:
Taxonomy of Remote Sensing Systems - Spatial Ground Resolution
- Ultra High: < 1m
- Very High: [1m, 4m]
- High: [4m, 10m]
- Medium: [10m, 50m]
- Low: [50m, 250m]
- Very Low: > 250m
The reference is:
Ehlers, M., Janowsky, R., Gähler, M., 2001. New remote sensing concepts for environmental monitoring. Proceedings of SPIE - The International Society for Optical Engineering.
The original paper is available at https://www.researchgate.net/publication/252130745_New_remote_sensing_concepts_for_environmental_monitoring
Follow my podcast: http://anchor.fm/tkorting
Subscribe to my YouTube channel: http://youtube.com/tkorting
The intro and the final sounds were recorded at my home, using an old clock that belonged to my grandmother.
Thanks for listening
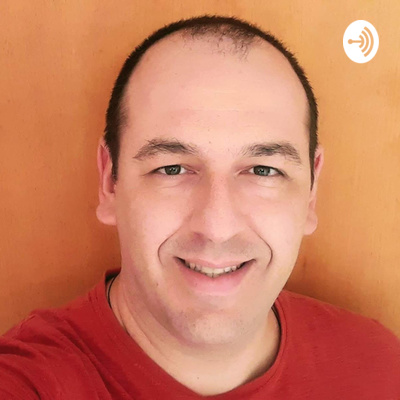
Is there an "Almost Perfect" agreement in a classification?
Landis, J.R. and Koch, G.G., 1977. The measurement of observer agreement for categorical data. Biometrics, pp.159-174.
According to Google Scholar, this paper has more than 53.000 citations (up to October, 2019). In my opinion this table has been used sometimes with a different purpose than the original paper, which, according to the authors, "have been illustrated with an example involving only two observers", and "these divisions are clearly arbitrary".
The original paper is available at www.jstor.org/stable/pdf/2529310.pdf
Follow my podcast: anchor.fm/tkorting
Subscribe to my YouTube channel: youtube.com/tkorting
The intro and the final sounds were recorded at my home, using an old clock that belonged to my grandmother.
Thanks for listening
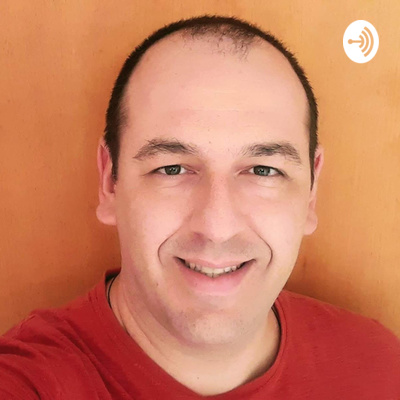
What is the origin of the term "Big Data"?
Follow my podcast: anchor.fm/tkorting
Subscribe to my YouTube channel: youtube.com/tkorting
The intro and the final sounds were recorded at my home, using an old clock that belonged to my grandmother.
Thanks for listening
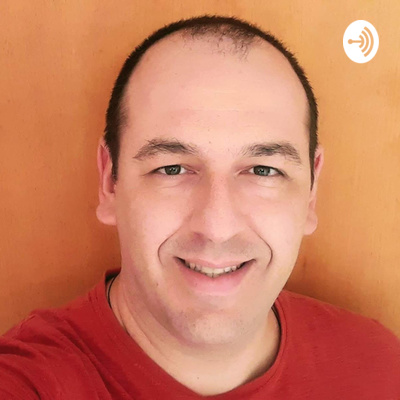
Unsupervised classification exists?
I use examples about remote sensing image classification and I discuss my opinion about the unsupervised algorithms, which are in fact similar to the supervised ones.
Take as one example the well known unsupervised K-Means algorithm. The analyst must inform a priori the most important parameter to run the algorithm, the K value.
I have a video about the K-Means algorithm.
Follow my podcast: anchor.fm/tkorting
Subscribe to my YouTube channel: youtube.com/tkorting
The intro and the final sounds were recorded at my home, using an old clock that belonged to my grandmother.
Thanks for listening
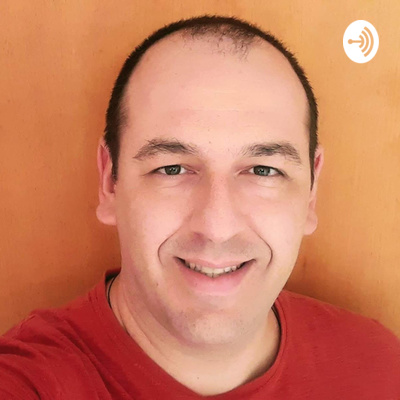
Waiting feedback - data mining, deep learning, remote sensing, image processing, pattern recognition
This is a first message to check if someone will find my podcast and will have interest on it. Waiting for feedback on remote sensing, image processing, data mining, deep learning, data augmentation, sample selection, articles, papers, etc.
Follow my podcast: http://anchor.fm/tkorting
Subscribe to my YouTube channel: http://youtube.com/tkorting
Thanks for listening